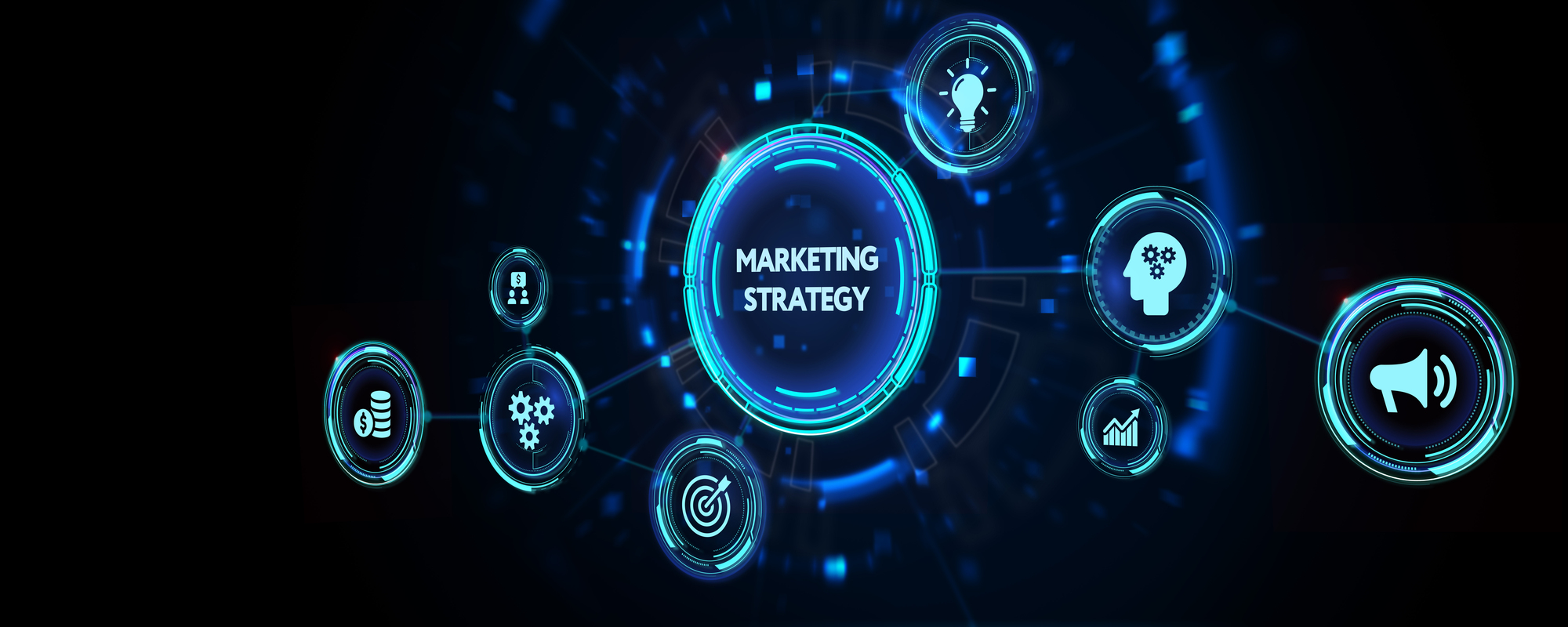
Understanding how to capture your audience’s attention is key to standing out. Two critical types of attention – endogenous and exogenous – offer unique opportunities for marketers to engage audiences strategically. Here’s a breakdown of each type and actionable strategies to harness their potential.
Endogenous Attention: Tapping Into Intentional Focus
Endogenous attention is deliberate and purpose-driven. It occurs when individuals consciously focus on something that aligns with their goals or interests, such as researching a product they plan to buy. This type of attention is internally motivated, requiring effort but offering sustained engagement when it resonates with personal objectives.
Key Characteristics:
- Driven by internal goals or motivations.
- Requires cognitive effort to maintain focus.
- Lasts longer when tied to personal relevance.
How to Capture Endogenous Attention:
- Deliver High-Value Content:
Provide content that directly addresses your audience’s needs or goals. Think in-depth guides, step-by-step tutorials, or insightful industry reports that empower them to achieve their objectives. - Optimize for Search Intent:
Use SEO strategies to ensure your content appears when users are actively looking for solutions related to your offerings. Align your messaging with the keywords and questions they’re searching for. - Personalize the Experience:
Tailor your communications based on individual preferences, behaviors, or past interactions. Personalized recommendations, targeted emails, or dynamic website content can make your message more relevant and engaging. - Craft Clear CTAs:
Use action-oriented calls-to-action (CTAs) that guide users toward achieving their goals. For instance, phrases like “Get Your Free Trial” or “Start Your Journey Today” resonate with their intent.