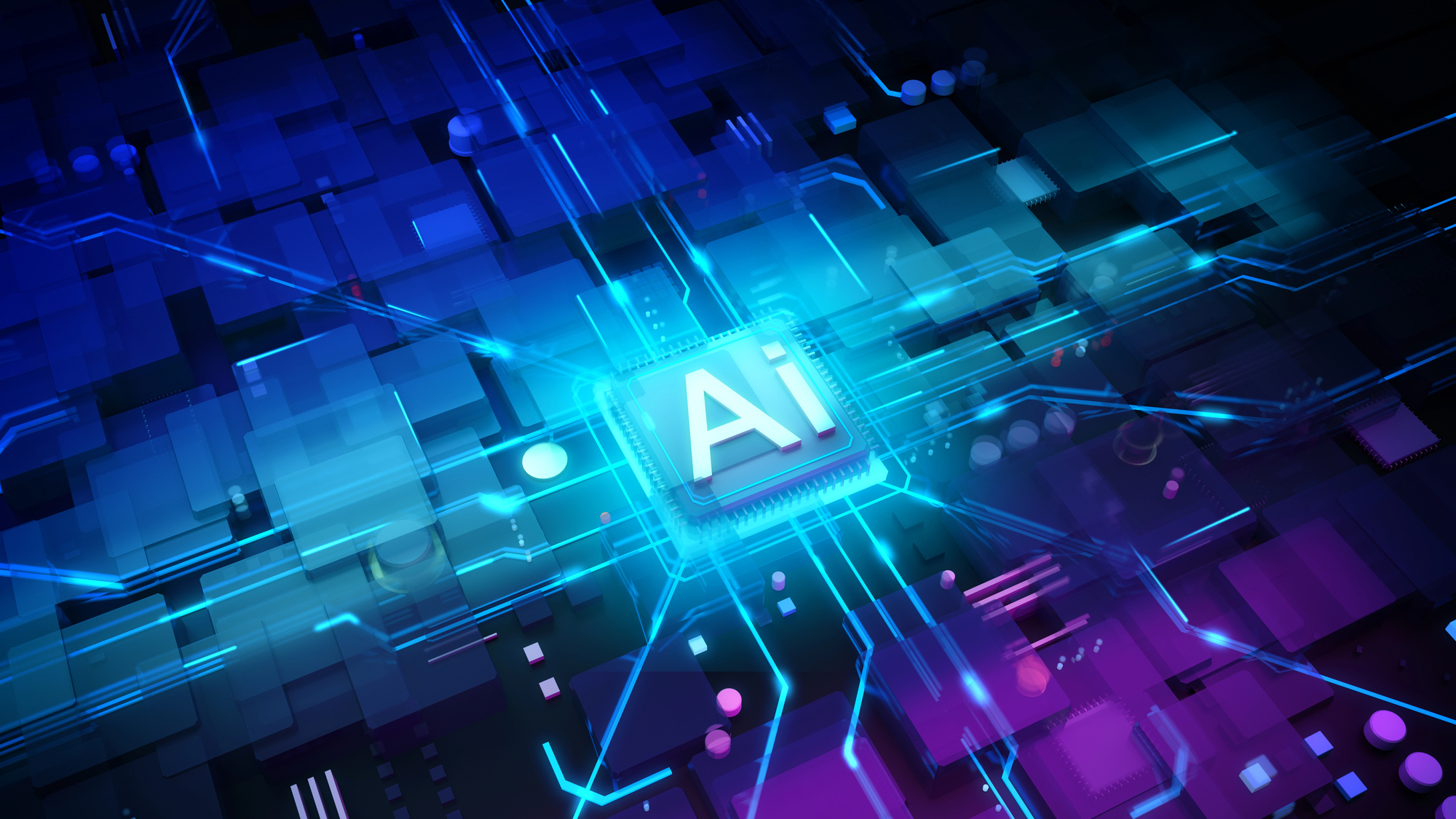
Introduction
As businesses strive to deliver more personalized and efficient services, the integration of AI into customer-facing applications has become paramount. Traditional AI models, while robust, often struggle with generating contextually accurate and up-to-date responses. Retrieval-Augmented Generation (RAG) and Inference-Time Processing address these limitations by combining the strengths of retrieval-based and generative AI models, enabling more accurate, relevant, and timely interactions.
Retrieval-Augmented Generation (RAG)
Retrieval-Augmented Generation (RAG) is a hybrid AI model that combines the capabilities of retrieval-based systems and generative models. RAG works by first retrieving relevant documents or information from a large corpus of data and then using a generative model to produce a response based on the retrieved information. This approach allows the model to generate more accurate and contextually relevant responses, especially in scenarios where up-to-date or domain-specific knowledge is required.
How RAG Works
- Retrieval Phase: The model queries a large database or knowledge base to retrieve relevant documents or information snippets. This retrieval is typically performed using dense vector representations and search techniques.
- Generation Phase: The retrieved information is then fed into a generative model along with the original query. The generative model synthesizes the information to produce a coherent and contextually appropriate response.
Benefits of RAG
Accuracy: By grounding responses in retrieved documents, RAG reduces the likelihood of generating incorrect or outdated information.
Relevance: The model can access and incorporate the most relevant information, leading to more precise and useful responses.
Scalability: RAG can be applied to large and dynamic datasets, making it suitable for businesses with extensive and ever-changing information repositories.
Inference-Time Processing
Inference-Time Processing refers to the techniques and optimizations applied during the execution (inference) of an AI model to improve its performance, accuracy, and efficiency.
Key Techniques in Inference-Time Processing
- Dynamic Filtering: Adjusting the model’s output based on real-time data or user context to ensure relevance and accuracy.
- Context-Aware Processing: Utilizing contextual information (e.g., user history, session data) to tailor responses and actions.
- Real-Time Data Integration: Incorporating live data feeds or updates into the inference process to provide the most current information.
Benefits of Inference-Time Processing
Real-Time Relevance: Ensures that the model’s outputs are always aligned with the latest data and user context.
Enhanced Performance: Optimizes the inference process to reduce latency and improve response times.
Adaptability: Allows the model to adapt to changing conditions and requirements dynamically.
Applications in Business Solutions
Intelligent Chatbots
Chatbots powered by RAG and Inference-Time Processing can deliver significantly improved customer interactions. By retrieving and synthesizing information from up-to-date knowledge bases, these chatbots can provide accurate and contextually relevant responses. Inference-Time Processing ensures that the chatbot adapts to the user’s context and provides real-time updates, enhancing the overall user experience.
Example:
A customer service chatbot for an ecommerce platform can use RAG to retrieve product information, policies, and FAQs. Inference-Time Processing allows the chatbot to dynamically adjust its responses based on the user’s browsing history, current cart contents, and real-time inventory data, providing a highly personalized and efficient service.
Enhanced Customer Experience Platforms
Integrating RAG and Inference-Time Processing into customer experience platforms enables businesses to offer more personalized and proactive support. These platforms can analyze customer interactions in real-time, retrieve relevant information, and generate tailored recommendations or solutions.
Example:
A financial services platform can use RAG to retrieve the latest market data, regulatory updates, and customer-specific information. Inference-Time Processing allows the platform to provide real-time investment advice, alert customers to relevant changes, and offer personalized financial planning based on current market conditions and individual goals.
Conclusion
Retrieval-Augmented Generation (RAG) and Inference-Time Processing represent significant advancements in AI technology, offering businesses the tools to create more accurate, relevant, and dynamic solutions. By leveraging these technologies, businesses can enhance their customer experience, improve operational efficiency, and stay competitive in an increasingly AI-driven world. As IT engineers, adopting and integrating RAG and Inference-Time Processing into your AI strategy will be crucial for developing next-generation business solutions that meet the evolving needs of your customers and stakeholders.
Call to Action
The potential of RAG and Inference-Time Processing is significant. A company should start by evaluating their current AI capabilities and identifying areas where these technologies can add value. I can assist your team to assess the current infrastructure and develop a roadmap to implement these advanced solutions.
The future of business innovation lies in the intelligent integration of AI, and the time to act is now. For leaders focused on driving digital growth and efficiency, I can partner with your team to guide that mission.
LinkedIn: https://www.linkedin.com/company/art-of-digital-commerce/
Contact: (760) 429-3800 | anna@artofdigitalcommerce.com