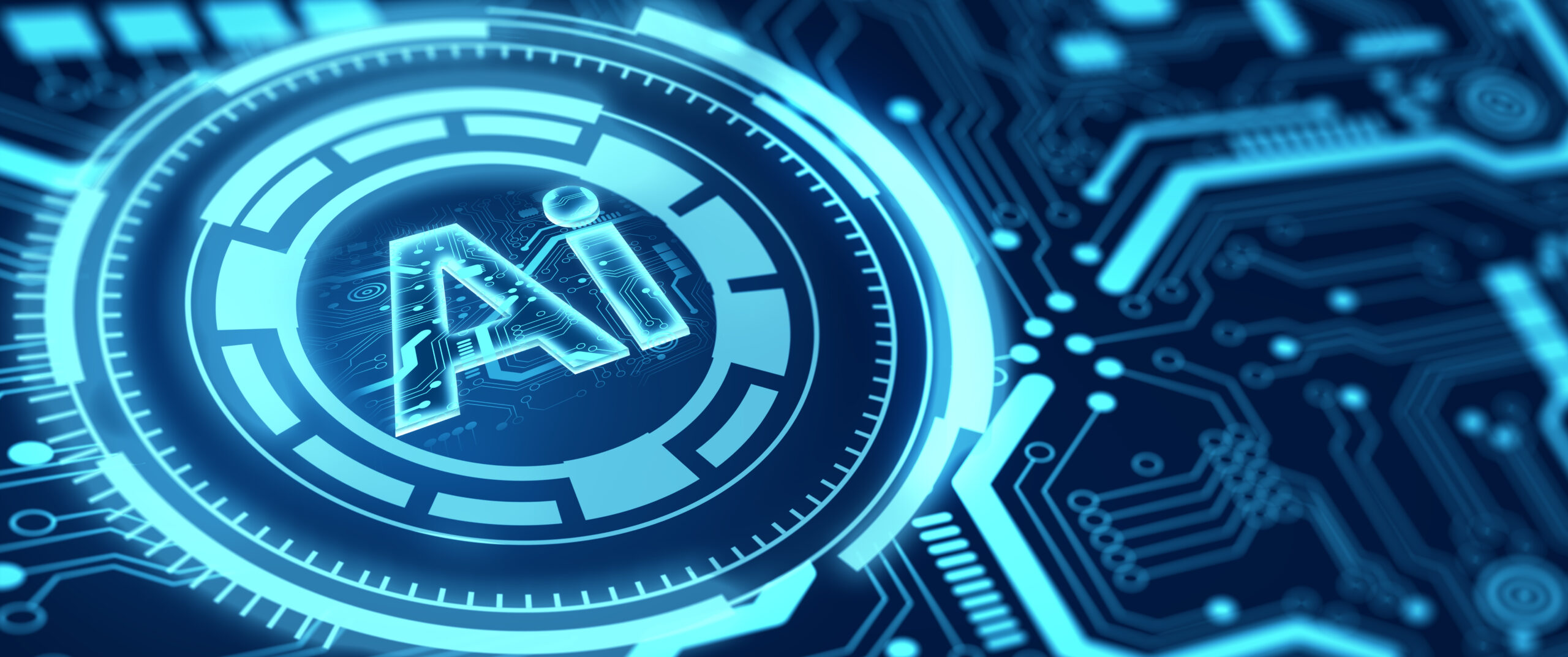
Recurrent Neural Networks (RNNs) and Convolutional Neural Networks (CNNs) are two types of artificial neural networks that are widely used in machine learning and artificial intelligence toolbox. They can play a significant role in a company’s digital transformation by enabling advanced data analysis, automation, and decision-making. Here’s an explanation of each and how they can be leveraged to create strategies and deliver on business objectives:
Recurrent Neural Networks (RNNs)
RNNs are a type of neural network designed to handle sequential data, where the order of data points matters. They are particularly useful for tasks involving time series data, natural language processing (NLP), and speech recognition.
RNNs excel in processing sequences, making them ideal for personalized marketing and customer behavior prediction.
Key Characteristics of RNNs
- Memory: RNNs have a “memory” that allows them to retain information from previous steps in a sequence. This makes them ideal for tasks where context or history is important.
- Sequential Processing: They process data one step at a time, making them suitable for tasks like predicting the next word in a sentence or forecasting stock prices.
Applications in Business
- Customer Sentiment Analysis: RNNs can analyze customer reviews, social media posts, or support tickets to gauge sentiment and identify trends.
- Demand Forecasting: By analyzing historical sales data, RNNs can predict future demand, helping optimize inventory and supply chain management.
- Chatbots and Virtual Assistants: RNNs power conversational AI tools that improve customer service and engagement.
- Fraud Detection: RNNs can detect unusual patterns in transaction data, helping to identify potential fraud in real time.
Strategic Value
- Personalization: RNNs enable personalized marketing and customer experiences by understanding user behavior over time.
- Operational Efficiency: By forecasting trends and automating repetitive tasks, RNNs help reduce costs and improve decision-making.
Convolutional Neural Networks (CNNs)
CNNs are a type of neural network designed to process grid-like data, such as images or videos. They are highly effective for tasks involving visual data and pattern recognition.
CNNs shine in analyzing images, enhancing product recommendations, and improving the user experience.
Key Characteristics of CNNs
- Feature Extraction: CNNs use convolutional layers to automatically detect important features (e.g., edges, shapes) in images or other grid data.
- Hierarchical Learning: They learn increasingly complex patterns, starting from simple features (e.g., lines) to more complex ones (e.g., objects).
Applications in Business
- Image and Video Analysis: CNNs can analyze visual data for quality control in manufacturing, facial recognition for security, or object detection in retail.
- Medical Imaging: In healthcare, CNNs can assist in diagnosing diseases by analyzing X-rays, MRIs, or CT scans.
- Retail and Ecommerce: CNNs power visual search tools, allowing customers to find products by uploading images.
- Autonomous Vehicles: CNNs are used to process visual data from cameras and sensors for navigation and obstacle detection.
Strategic Value
- Innovation: CNNs enable businesses to develop cutting-edge products and services, such as augmented reality (AR) or smart surveillance systems.
- Customer Experience: Visual search and recommendation systems enhance the shopping experience, driving sales and customer loyalty.
- Automation: CNNs automate tasks like quality inspection, reducing human error and operational costs.
How RNNs and CNNs Support Digital Transformation
Data-Driven Decision Making
– Both RNNs and CNNs extract insights from large datasets, enabling leaders to make informed, data-driven decisions.
– For example, RNNs can predict customer churn, while CNNs can analyze customer behavior through visual data.
Automation and Efficiency
– These technologies automate repetitive tasks, freeing up resources for strategic initiatives.
– For instance, RNNs can automate customer support through chatbots, and CNNs can automate quality control in manufacturing.
Enhanced Customer Experiences
– By leveraging RNNs and CNNs, businesses can deliver personalized and seamless customer experiences.
– Examples include personalized product recommendations (RNNs) and visual search capabilities (CNNs).
Innovation and Competitive Advantage
– RNNs and CNNs enable businesses to innovate and stay ahead of competitors by adopting AI-driven solutions.
– For example, CNNs can power AR applications, while RNNs can enable real-time language translation for global markets.
Scalability
– These models can scale with the business, handling increasing amounts of data and complexity as the company grows.
Steps to Incorporate RNNs and CNNs into Your Digital Transformation Roadmap
- Identify Key Business Challenges
Determine where RNNs or CNNs can add value, such as improving customer engagement, optimizing operations, or enhancing product offerings.
- Invest in Data Infrastructure
Ensure you have the necessary data collection, storage, and processing capabilities to support AI models.
- Upskill or Partner for AI Expertise
Develop in-house AI capabilities or partner with technology providers to implement RNNs and CNNs effectively.
- Pilot and Scale
Start with pilot projects to test the effectiveness of RNNs and CNNs in specific use cases, then scale successful initiatives across the organization.
- Measure Impact
Continuously monitor the performance of AI-driven solutions and adjust strategies to maximize ROI.
Conclusion
Integrating these advanced neural networks into your digital transformation strategy can unlock numerous benefits. A study by McKinsey found that personalization can drive a 10-30% increase in ecommerce revenue.
On the other hand, CNNs can automatically categorize and tag product images, making it easier for customers to find what they’re looking for. This efficiency not only saves time but also boosts sales conversions.
In my own experience, implementing CNNs in a D2C digital brand’s product listing improved search functionality with a 25% increase in click-through rates within a few months.
Let’s discuss how RNNs and CNNs can work for your business and dive deeper into actionable strategies for implementing these technologies in your operation.
The future of business innovation lies in the intelligent integration of AI, machine learning, and emerging technologies. The time to act is now. For leaders focused on driving digital growth and efficiency, I can partner with your team to guide that mission.
LinkedIn: https://www.linkedin.com/company/art-of-digital-commerce/
Contact: (760) 429-3800 | anna@artofdigitalcommerce.com